
Nvidia achieved a remarkable milestone by joining the 3-trillion-dollar market valuation club in June 2024, surpassing industry giants like Apple and Microsoft. This extraordinary rise is attributed to Nvidia’s stronghold in the GPU and AI hardware sectors. However, it is vital to recognize that Nvidia is not the sole player in the production of chips catering to the expanding AI workload demands. Several companies, including Intel, Google, and Amazon, are actively developing custom silicon designed for training and inference applications in AI.
AMD
Among the notable contenders challenging Nvidia in the AI hardware landscape is AMD. The company has made significant strides with its high-performance AI accelerators, particularly the Instinct MI300X, which launched in December 2023. Despite industry analysts estimating Nvidia’s market share at approximately 70% to 90%, AMD is working diligently to enhance its position.
The Instinct MI300X is designed specifically for AI workloads and high-performance computing (HPC). AMD asserts that the MI300X outperforms Nvidia’s H100 by a factor of 1.6 in inference tasks and shows comparable performance in training scenarios. Furthermore, the MI300X boasts an impressive capacity of up to 192GB of High-Bandwidth Memory (HBM3), significantly exceeding the 80GB capacity offered by the Nvidia H100. In terms of memory bandwidth, the MI300X claims a rate of up to 5.3 TBPS, again outpacing the H100, which provides 3.4 TBPS.
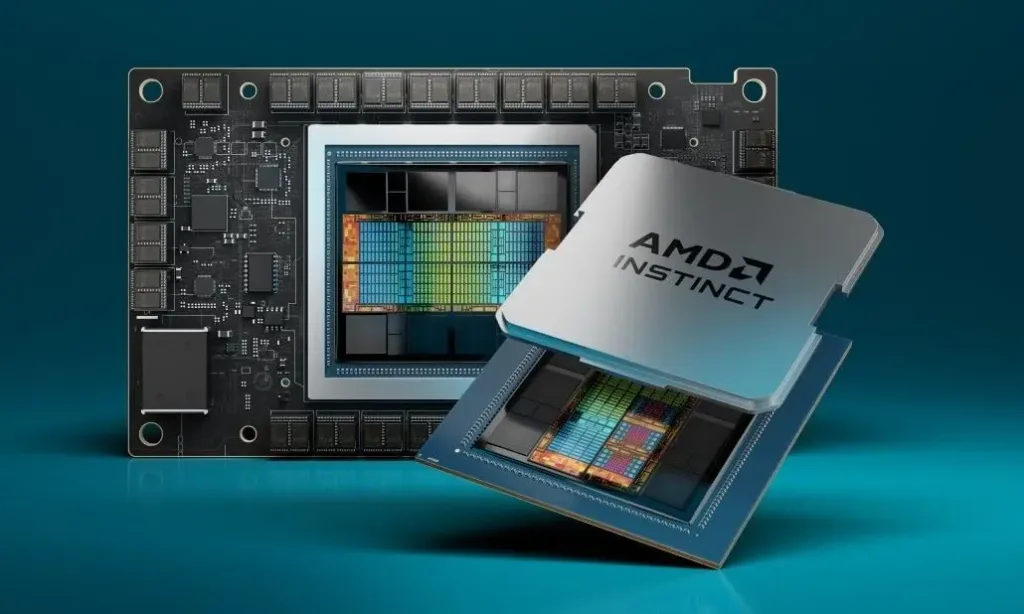
AMD is indeed making significant efforts to challenge Nvidia’s dominance in the AI hardware sector. However, establishing itself as a substantial competitor is a complex journey, particularly when it comes to software solutions. Nvidia’s competitive advantage lies mainly in its CUDA platform, which enables developers to harness the full capabilities of Nvidia GPUs for accelerated parallel processing.
CUDA is rich with numerous libraries, software development kits (SDKs), toolkits, compilers, and debugging tools, and it integrates seamlessly with major deep learning frameworks such as PyTorch and TensorFlow. Having been established for nearly two decades, CUDA has become the standard among developers, who are generally more familiar with Nvidia’s ecosystem, especially in machine learning applications. Nvidia has not only built a robust platform but also fostered a vibrant community around CUDA, backed by extensive documentation and training materials to support developers.
AMD needs to unify its software platform and attract ML researchers and developers by improving ROCm documentation and support. Notably, major companies like Microsoft, Meta, OpenAI, and Databricks are already using MI300X accelerators with ROCm, indicating progress.
Intel
Despite some analysts dismissing Intel in the AI chip market, the company remains a leader in inferencing with its CPU-based Xeon servers. Intel’s recent launch of the Gaudi 3 AI accelerator, an ASIC designed specifically for this purpose, enhances its capabilities for both training and inference of Generative AI workloads.
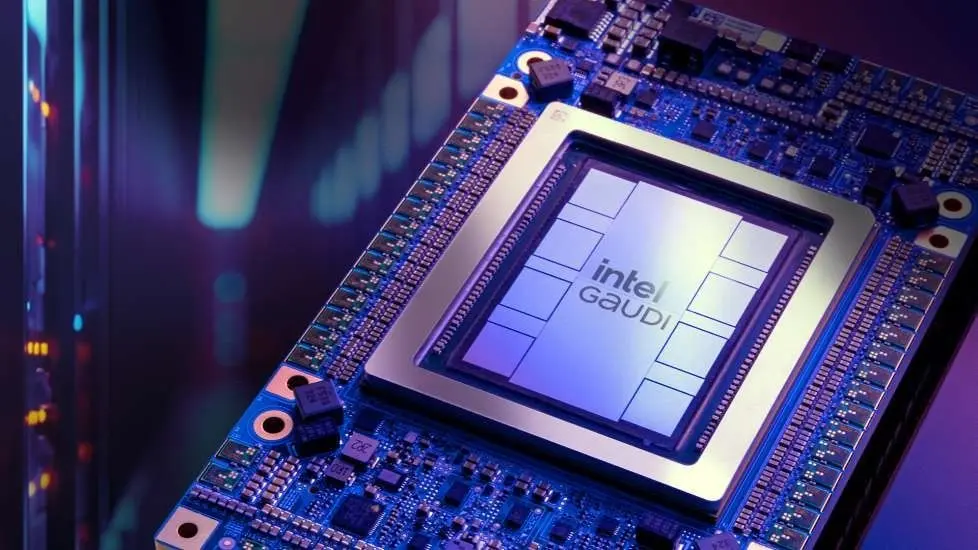
Intel claims the Gaudi 3 AI accelerator is 1.5 times faster in training and inference than the Nvidia H100. Its Tensor Processor Cores (TPC) and MME Engines are optimized for the matrix operations essential for deep learning.
On the software side, Intel is adopting an open-source approach with OpenVINO and its own software stack. The Gaudi software suite includes frameworks, tools, drivers, and libraries, and it supports popular frameworks like PyTorch and TensorFlow. Regarding Nvidia’s CUDA, Intel CEO Pat Gelsinger recently stated:
The entire industry seems driven to disrupt the CUDA market, viewing the CUDA advantage as minor and easily overcome.
Intel, along with Google, Arm, Qualcomm, Samsung, and others, has formed the Unified Acceleration Foundation (UXL) to develop an open-source alternative to Nvidia’s proprietary CUDA software platform. The goal is to create a silicon-agnostic platform that allows models to be trained and run on any chip, preventing developers from being locked into Nvidia’s ecosystem.
The future remains uncertain, but Intel’s effort to challenge CUDA is underway.
Google stands out as an AI giant not reliant on Nvidia. Since 2015, it has been developing its in-house TPU (Tensor Processing Unit) based on ASIC design. The powerful TPU v5p is 2.8 times faster than the Nvidia H100 for training AI models and is highly efficient for inference. The sixth-generation Trillium TPU offers even greater capabilities. Google employs its TPUs for training, fine-tuning, and inference.
At the Google Cloud Next 2024 event, Patrick Moorhead, Founder and CEO at Moor Insights & Strategy, confirmed that Google’s Gemini model was entirely trained on the TPU, a significant milestone, as it is also currently used for inference.
Google provides its TPU through Google Cloud, supporting a variety of AI workloads. Notably, Apple’s AI models were trained on Google’s TPU, establishing Google as a formidable rival to Nvidia. With its custom silicon, Google outperforms other chip makers in both training and inference.
Unlike Microsoft, Google is not overly reliant on Nvidia. It also introduced the Axion processor, an Arm-based CPU that offers unmatched efficiency for data centers and supports CPU-based AI training and inference.
Additionally, Google excels in software support, seamlessly integrating frameworks like JAX, Keras, PyTorch, and TensorFlow.
Amazon
On the other hand, Amazon operates AWS (Amazon Web Services), providing cloud-computing platforms for businesses. To address AI workloads, Amazon has developed two custom ASIC chips: AWS Trainium for deep-learning training capable of handling up to 100 billion parameters, and AWS Inferentia for AI inference.
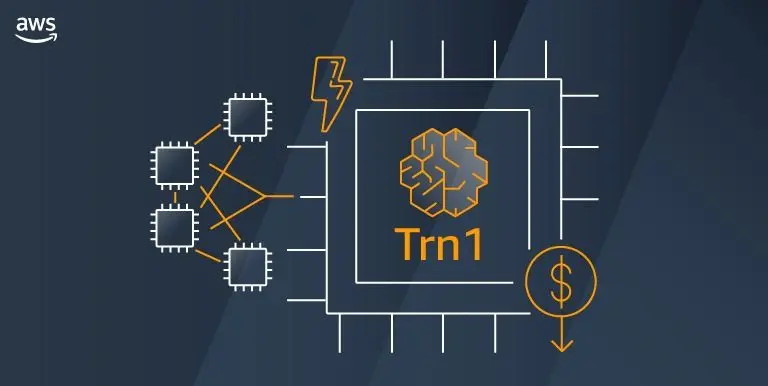
AWS custom chips are designed to provide low cost and high performance, as Amazon scales its efforts to establish a presence in the AI hardware market. The company also offers the AWS Neuron SDK, which integrates popular frameworks like PyTorch and TensorFlow.
Microsoft
Like Google, Microsoft is increasing its focus on custom silicon. In November 2023, it launched the MAIA 100 chip for AI workloads and the Cobalt 100, an Arm-based CPU for Azure cloud infrastructure, aiming to reduce its dependence on Nvidia.
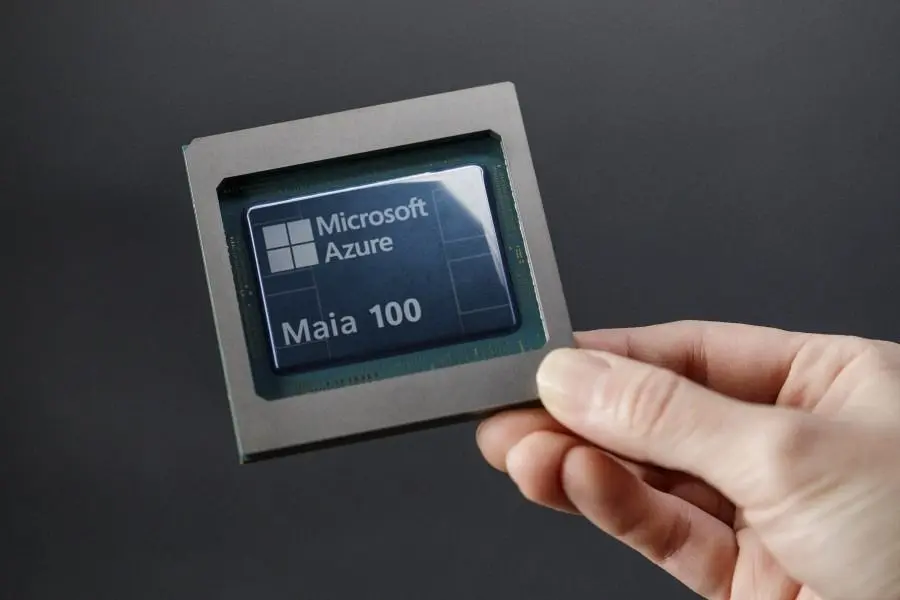
The MAIA 100 chip, built on an ASIC design, is specifically intended for AI training and inference, and it is currently being tested for GPT-3.5 Turbo inference. Microsoft maintains strong partnerships with Nvidia and AMD for its cloud infrastructure.
The future of these relationships remains uncertain as Microsoft and other companies begin to deploy their custom silicon more widely.
Qualcomm
Qualcomm launched its Cloud AI 100 accelerator in 2020 for AI inferencing, but it did not gain the expected traction. In November 2023, the company introduced the Cloud AI 100 Ultra, a custom-built ASIC designed for generative AI applications. This chip can manage 100 billion parameter models on a single card with a thermal design power (TDP) of just 150W.
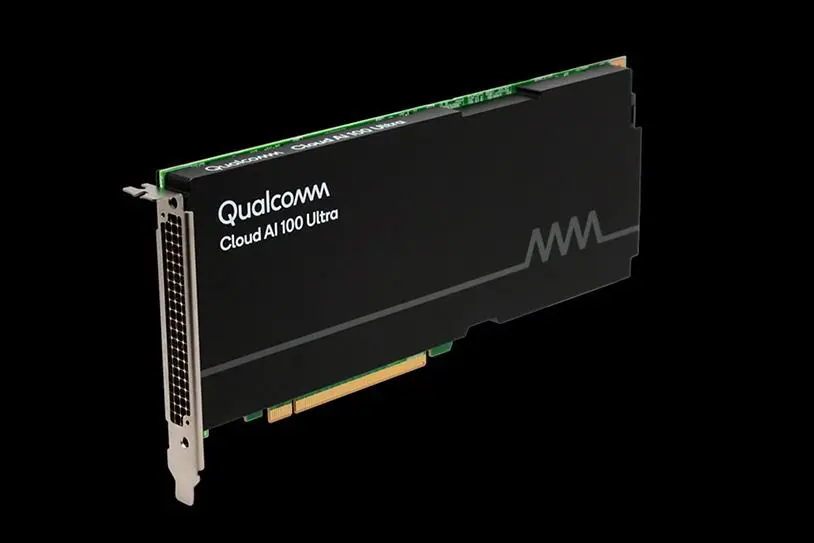
Qualcomm has also developed its own AI stack and cloud AI SDK, focusing primarily on inferencing rather than training. The Cloud AI 100 Ultra promises exceptional power efficiency, delivering up to 870 TOPS for INT8 operations.
Hewlett Packard Enterprise (HPE) is utilizing the Qualcomm Cloud AI 100 Ultra to enhance generative AI workloads on its servers. Additionally, Qualcomm has partnered with Cerebras to offer end-to-end model training and inference on a unified platform.
Cerebras
Cerebras, a startup focused on training large-scale AI systems, has developed the Wafer-Scale Engine 3 (WSE-3), a massive processor capable of handling models with up to 24 trillion parameters—ten times the size of GPT-4.
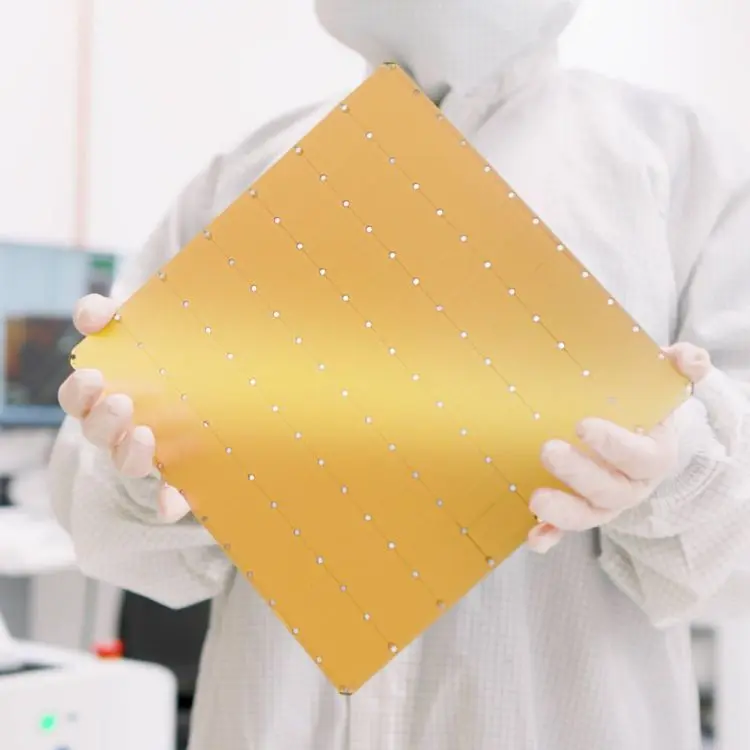
The WSE-3 features an impressive 4 trillion transistors, utilizing nearly the entire wafer, which eliminates the need for multiple interconnected chips and memory. This design reduces power consumption by minimizing data movement between components, outperforming Nvidia’s advanced Blackwell GPUs in petaflops per watt.
Targeted at large corporations seeking to build powerful AI systems without relying on distributed computing, Cerebras counts customers like AstraZeneca, GSK, The Mayo Clinic, and major US financial institutions among its clients.
Additionally, the company recently launched its Cerebras Inference API, which delivers exceptional performance for Llama 3.1 models with 8 billion and 70 billion parameters.
Groq
Groq made waves in the AI industry this year with its LPU (Language Processing Unit) accelerator, achieving a consistent output of 300 to 400 tokens per second while running the Llama 3 70B model. It is now the second-fastest AI inferencing solution available to developers for production applications, following Cerebras.
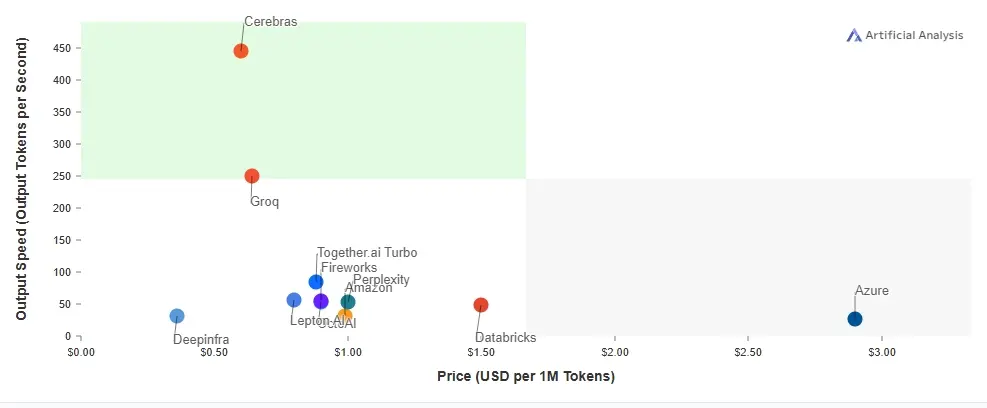
Designed by former Google TPU engineers, Groq’s ASIC chip is purpose-built for generative AI applications and enables large-scale parallelism. Operating AI models on Groq’s LPU is more cost-effective than using Nvidia GPUs, and while it performs well with smaller models, its efficiency with 500 billion or trillion-parameter models remains to be tested.
Final thoughts
In summary, several chipmakers beyond Nvidia are competing in the AI hardware space. While SambaNova offers training-as-a-service, it lacks quantifiable benchmarks for assessment. Meanwhile, Tenstorrent is shifting towards RISC-V-based IP licensing for its chip designs.
Overall, the AI industry is increasingly focusing on custom silicon and in-house AI accelerators. Although Nvidia remains the preferred choice for training due to the widespread adoption of CUDA, the landscape may change as more specialized accelerators evolve. For inference, several current solutions already surpass Nvidia’s performance.
0 Comments