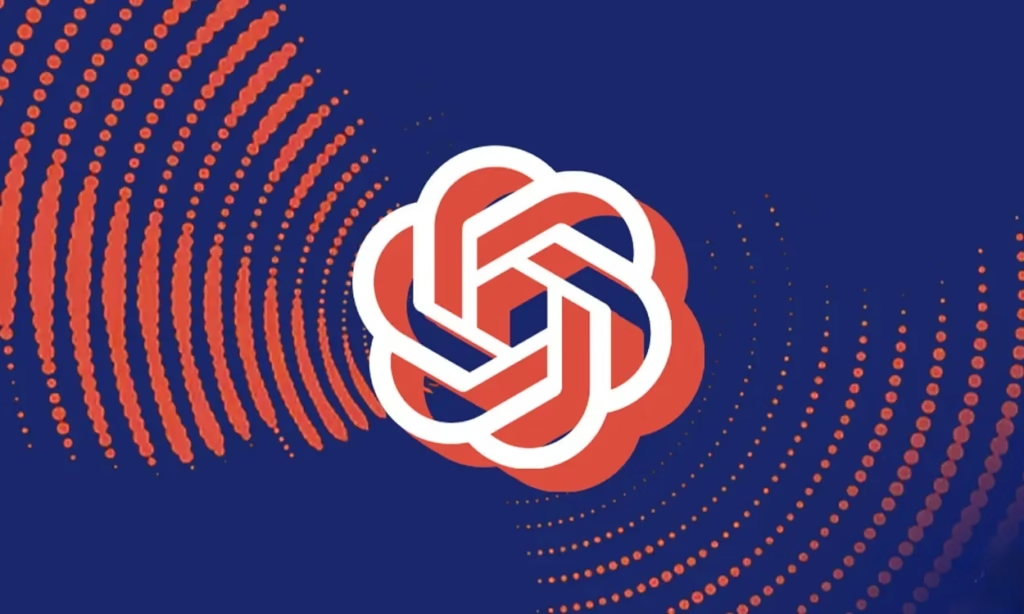
While OpenAI chief Sam Altman hypes AGI as being just around the corner, new reports indicate that LLM scaling may have reached its limit. The prevailing belief in AI has been that increasing model size, data, and compute power leads to greater intelligence.
Ilya Sutskever, former chief scientist at OpenAI and founder of Safe Superintelligence Inc., has long championed model scaling as the key to unlocking intelligence. However, in response to Reuters, Sutskever now acknowledges that “results from scaling up pre-training the phase where an AI model learns language patterns and structures from vast amounts of unlabeled data have plateaued.”
In a shift of perspective, Sutskever emphasizes the importance of what we scale: “The 2010s were the age of scaling. Now, we’re back in the age of wonder and discovery once again. Everyone is looking for the next breakthrough. Scaling the right thing matters more now than ever.”
This shift in approach is why OpenAI introduced its new series of ’01’ reasoning models on ChatGPT, which emphasize scaling during inference. Research shows that allowing AI models more time to “think” and refine their responses leads to significantly better outcomes. As a result, companies are now prioritizing test-time compute allocating more resources during inference to enhance the quality of the final output.
Recently, The Information reported a strategic shift at OpenAI after its upcoming “Orion” model failed to deliver the anticipated improvements. While the leap from GPT-3.5 to GPT-4 was substantial, employees who tested Orion found that its gains over GPT-4 were minimal. In tasks such as coding, Orion doesn’t surpass previous GPT models.
OpenAI is now shifting its focus to inference scaling as a key strategy for enhancing model performance on ChatGPT. Noam Brown, a researcher at OpenAI, states that scaling during inference leads to significant improvements in model performance.
Recently, he tweeted, “OpenAI’s o1 thinks for seconds, but we aim for future versions to think for hours, days, even weeks. Inference costs will be higher, but what cost would you pay for a new cancer drug? For breakthrough batteries? For a proof of the Riemann Hypothesis? AI can be more than chatbots.”
Google and Anthropic are also exploring inference scaling as a technique to enhance model performance. However, François Chollet, a researcher at Google, argues that scaling LLMs alone won’t result in generalized intelligence. Similarly, Yann LeCun, chief AI scientist at Meta, states that LLMs are insufficient for achieving AGI.
As companies exhaust available data for training larger models, they are seeking innovative approaches to boost LLM performance. Whether AGI is truly just around the corner or merely hype remains to be seen.