
During the Google I/O 2023 conference in June, Google unveiled Gemini, its highly capable AI model, offering a sneak peek into its potential. By the end of 2023, Google made the Gemini AI models accessible to the public, marking it as a monumental stride, the “Gemini era” for the company. However, the question arises: What precisely is Google’s Gemini AI, and could it challenge the reigning champion, GPT-4? To delve into these inquiries, let’s explore our comprehensive breakdown of the Gemini AI models.
What is Google Gemini AI?
Gemini emerges as the latest and most advanced large language model (LLM) developed by Google’s DeepMind team, based in London. Positioned as the successor to the PaLM 2 model, crafted within Google’s in-house AI division, Gemini represents the inaugural full-scale release of an AI system by the DeepMind team to the public.
A pivotal moment in this development was the merger of Google’s Google Brain division with the DeepMind team in April 2023. This amalgamation aimed to forge a robust model capable of rivaling OpenAI’s most formidable creations, culminating in the birth of Gemini through this collaborative endeavor.
Gemini AI stands out in its multimodal capabilities, distinguishing itself from both OpenAI’s GPT-4 and its predecessor, the PaLM 2 model. While PaLM 2 did support image analysis, it relied on external tools like Google Lens and semantic analysis to interpret data from uploaded images. This approach served as a makeshift method by Google to incorporate image support into Bard.
In contrast to GPT-4, which also possesses multimodal capabilities, Gemini AI takes a different approach. In our detailed exploration of the upcoming GPT-5 model, we revealed that GPT-4 isn’t a singular dense model. Instead, it operates on a “Mixture of Experts” architecture, comprising 16 distinct models interconnected for various tasks. For tasks such as image analysis, generation, and voice processing, GPT-4 employs separate models like GPT-4 Vision, DALL·E, Whisper, among others.
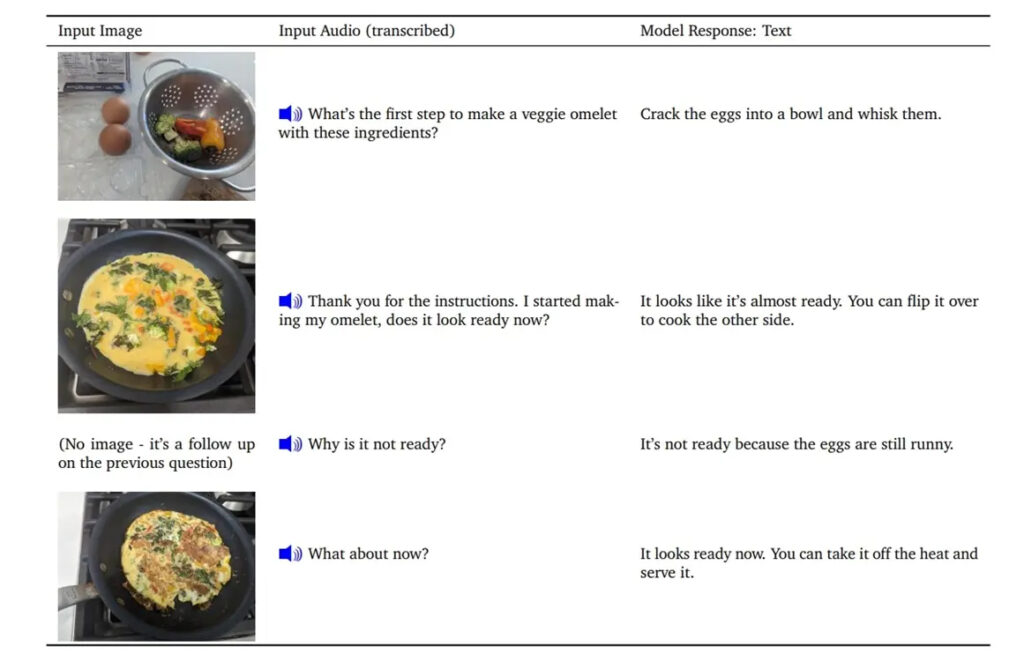
The distinctive feature of Google’s Gemini lies in its status as a “natively multimodal AI model,” meticulously crafted from the ground up to encompass text, image, audio, video, and code within a unified training framework, resulting in a formidable AI system.
Thanks to Gemini’s inherent multimodal design, it possesses the remarkable ability to process information seamlessly across diverse modalities simultaneously.
For end users, the advantages of a native multimodal AI system are extensive, as we’ll delve into shortly. But first, let’s explore the intricacies of Gemini’s multimodal capabilities.
Gemini AI is Truly Multimodal
Indeed, differentiating Gemini AI from other multimodal models is best illustrated through an example, such as audio processing. OpenAI’s Whisper v3, a prominent speech recognition model, excels in recognizing multilingual speech, identifying languages, transcribing speech, and even performing translations. However, Whisper falls short when it comes to understanding the subtle nuances of audio, such as identifying tone, mood, or subtle variations in pronunciation.
For instance, while someone may convey varying emotions while saying “hello,” Whisper merely transcribes the audio without capturing the mood or nuances. In contrast, Gemini AI stands out by processing raw audio signals end-to-end, enabling it to capture these intricate details and nuances. Google’s AI model can discern emotions, differentiate pronunciations in various languages, and transcribe with nuanced annotations, making Gemini a more adept and capable multimodal system in capturing the intricate aspects of audio data.
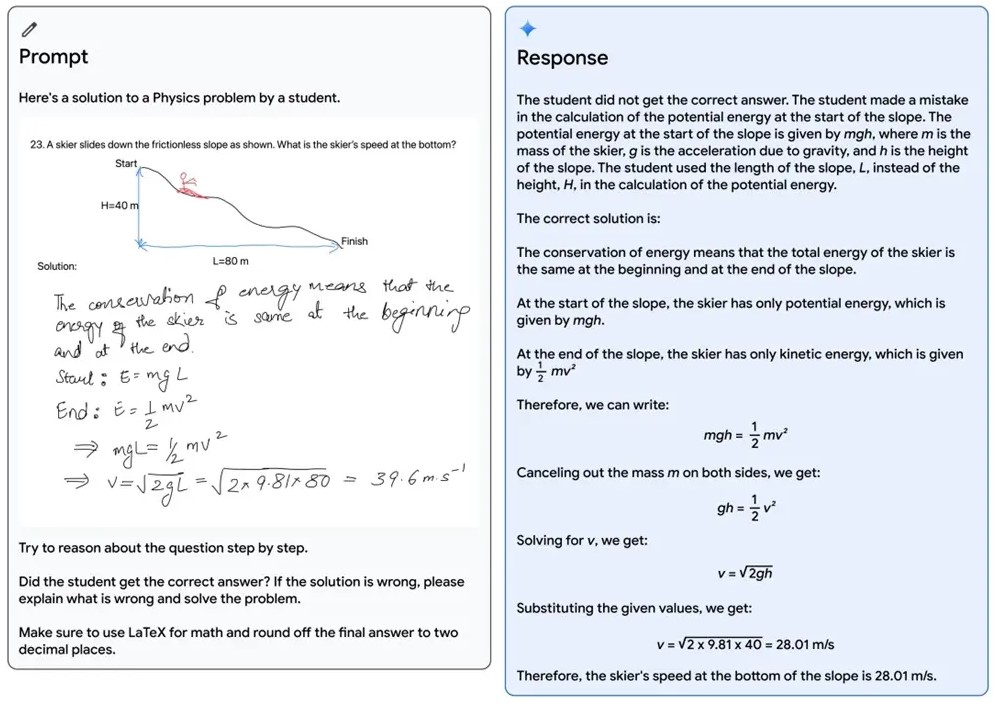
Gemini’s prowess extends to both image analysis and generation, possibly leveraging Imagen 2 capabilities. In visual analysis, Gemini’s capabilities are extensive and impressive. It excels in establishing connections between images, making educated guesses about movies based on stills, transforming images into code, comprehending the surrounding environment, interpreting handwritten texts, elucidating reasoning in mathematical and physical problems, and much more. This remains true despite speculation that Google faked the Gemini AI demo.
Furthermore, Gemini demonstrates competence in processing and comprehending video content. In the realm of coding, Gemini AI boasts support for numerous programming languages, encompassing popular ones like Python, Java, C++, and Go. Its coding proficiency surpasses that of PaLM 2, particularly in tackling intricate coding challenges. Gemini achieves a commendable 75% success rate in solving Python functions on the first attempt, whereas PaLM 2 could only manage 45%. Moreover, when prompted with debug input, Gemini’s solve rate surpasses 90%, highlighting its robust problem-solving capabilities.
Google has developed a specialized iteration of Gemini tailored specifically for advanced code generation, known as AlphaCode 2. This specialized version demonstrates exceptional proficiency in competitive programming, efficiently solving highly challenging problems involving intricate mathematics and theoretical computer science. When pitted against human competitors, AlphaCode 2 outperforms an impressive 85% of participants engaged in competitive programming.
In essence, Google’s Gemini emerges as a remarkable multimodal AI system adept at various applications, including textual generation and reasoning, image analysis, code generation, audio processing, and video comprehension, showcasing its versatility across diverse use cases.
Gemini AI Comes in Three Flavors
Google has unveiled the Gemini AI in three distinct variants – Ultra, Pro, and Nano – while keeping the specific parameter sizes undisclosed. Positioned as Google’s largest and most capable model akin to GPT-4, the Gemini Ultra variant boasts a comprehensive set of multimodal capabilities. According to the company, the Ultra model is tailored for handling highly intricate and exceedingly demanding tasks.

However, the Gemini Ultra model remains unreleased as of now. Google has indicated that Ultra will undergo stringent trust and safety evaluations, and it is slated for an early launch next year, intended for developers and enterprise clientele.
Google plans to introduce Bard Advanced, enabling consumers to access Gemini Ultra’s complete multimodal capabilities early next year. This launch may also grant users access to AlphaCode 2.
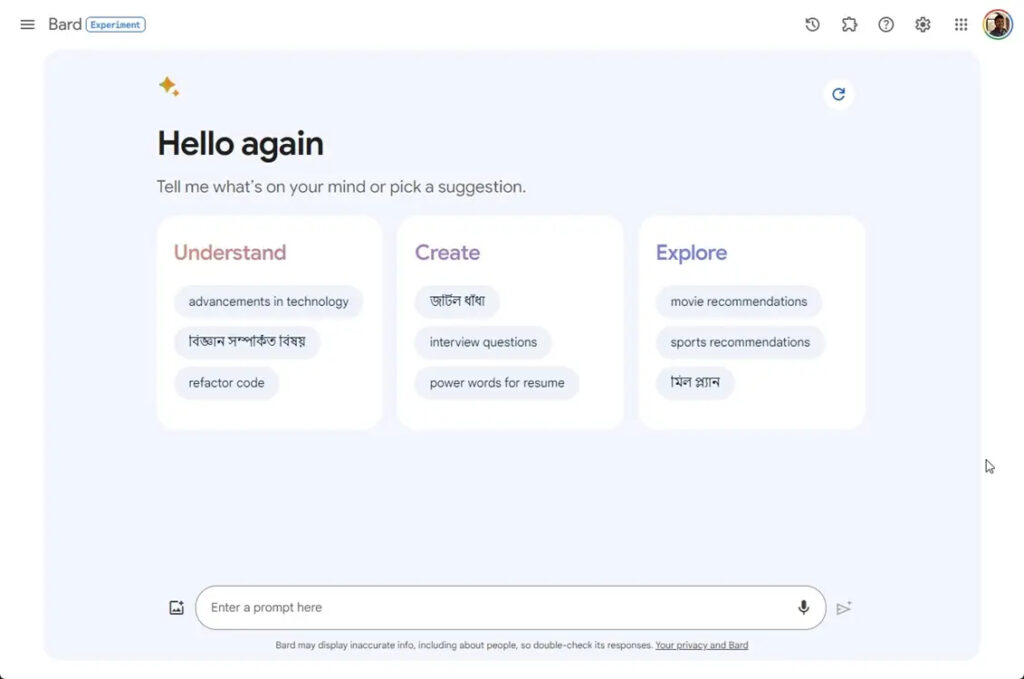
Gemini Pro is currently operational on the Google Bard alternative for ChatGPT, and the transition from PaLM 2 to Gemini Pro is slated for completion by the end of December.
Crafted for a diverse array of tasks, the Pro model surpasses OpenAI’s GPT-3.5 on multiple benchmarks, as detailed below. Additionally, Google has rolled out APIs for both text and vision models of the Gemini Pro variant.
At present, the Gemini Pro model is available solely in English across more than 170 countries worldwide. However, Bard will soon receive multimodal support and extend language coverage, aiming to integrate Gemini Pro.
Google has plans to integrate Gemini into additional products like Search, Chrome, Ads, and Duet AI in the upcoming months.
As for the Nano variant, it has already debuted on the Pixel 8 Pro and will expand to other Pixel devices. Designed for on-device, private, and personalized AI experiences on smartphones, Nano powers features such as Summarize in the Recorder app and Smart Reply in Gboard, initially focusing on WhatsApp, Line, and KakaoTalk. Support for other messaging apps is scheduled for inclusion in the early part of the coming year.
Google Gemini AI is Efficient to Run
The inherent advantages of a native multimodal AI system are manifold, primarily attributed to its speed, efficiency, and scalability when compared to alternative models. Notably, OpenAI’s GPT-4 has faced challenges regarding speed, prompting the company to halt its ChatGPT Plus subscription due to hardware requirements. The approach of running separate text, vision, and audio models and integrating them in an inefficient manner contributes to increased infrastructure costs, ultimately impacting the user experience.
Google emphasizes in its blog post that Gemini operates on its highly efficient TPU systems, notably v4 and v5e, offering significant speed and scalability advantages. The utilization of AI accelerators for the Gemini model proves faster and more cost-effective in comparison to the older PaLM 2 model. Consequently, leveraging a native multimodal model enables Google to efficiently cater to millions of users while maintaining lower compute costs.
Gemini Ultra vs GPT-4: Benchmarks
The release of Gemini has prompted a closer look at benchmark numbers to assess its performance compared to OpenAI’s GPT-4. According to Google’s claims, Gemini Ultra surpasses the GPT-4 model in 30 out of 32 benchmark tests typically used to gauge Large Language Model (LLM) performance. Notably, Gemini Ultra achieved a notable 90.04% score on the MMLU benchmark test, outperforming GPT-4, which scored 86.4%. Impressively, Gemini Ultra even surpasses human experts, scoring 89.8% on the MMLU benchmark.
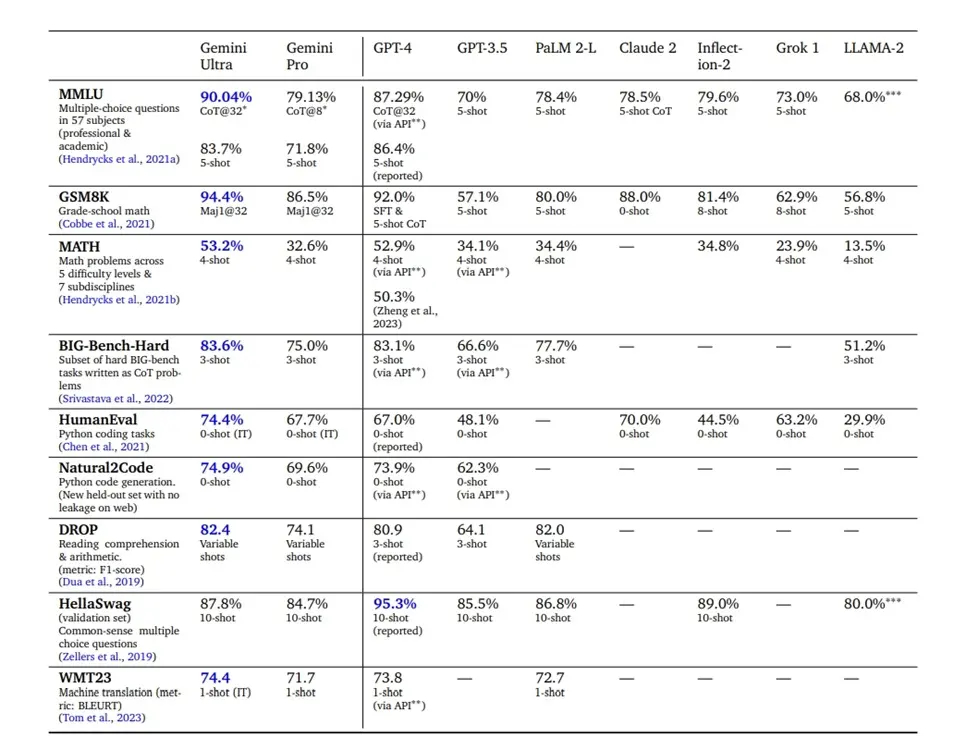
However, scrutiny has arisen regarding Gemini Ultra’s MMLU benchmark score. Google’s achievement of a 90.04% score with CoT@32 (Chain-of-Thought) prompting to secure accurate responses has garnered criticism. When employing the standard 5-shot prompting, Gemini Ultra’s score decreases to 83.7%, while GPT-4 maintains a score of 86.4%, retaining its lead as the highest scorer in the MMLU test.
The comparative analysis between Gemini Ultra and GPT-4 showcases distinctive performances across various benchmarks. While this doesn’t undermine Gemini Ultra’s capabilities, it underscores the necessity for more precise and refined prompting methods to extract accurate responses from the model.
In the standard 5-shot prompting scenario, Gemini Ultra achieves a score of 83.7%, slightly trailing behind GPT-4, which maintains its lead with a score of 86.4% in the MMLU test.
Examining other benchmarks, Gemini Ultra excels in the HumanEval (Python code generation) test, scoring 74.4% compared to GPT-4’s 67.0%. However, in the HellaSwag test, assessing commonsense reasoning, Gemini Ultra (87.8%) falls short of GPT-4 (95.3%). Conversely, in the Big-Bench Hard benchmark, assessing challenging multi-step reasoning tasks, Gemini Ultra (83.6%) narrowly outperforms GPT-4 (83.1%).
In the realm of multimodal tests, Gemini Ultra emerges triumphant in most comparisons against GPT-4V (Vision). Across various assessments, Gemini Ultra consistently leads GPT-4V. For instance, in the MMMU test, Gemini Ultra achieves a score of 59.4% compared to GPT-4V’s 56.8%. Additionally, in natural image understanding (VQAv2 test), Gemini Ultra scores 77.8%, surpassing GPT-4V’s 77.2%. In tests like OCR on natural images (TextVQA), document understanding (DocVQA), and Infographic understanding, Gemini Ultra secures scores of 82.3%, 90.9%, and 80.3%, respectively, outperforming GPT-4V’s scores.
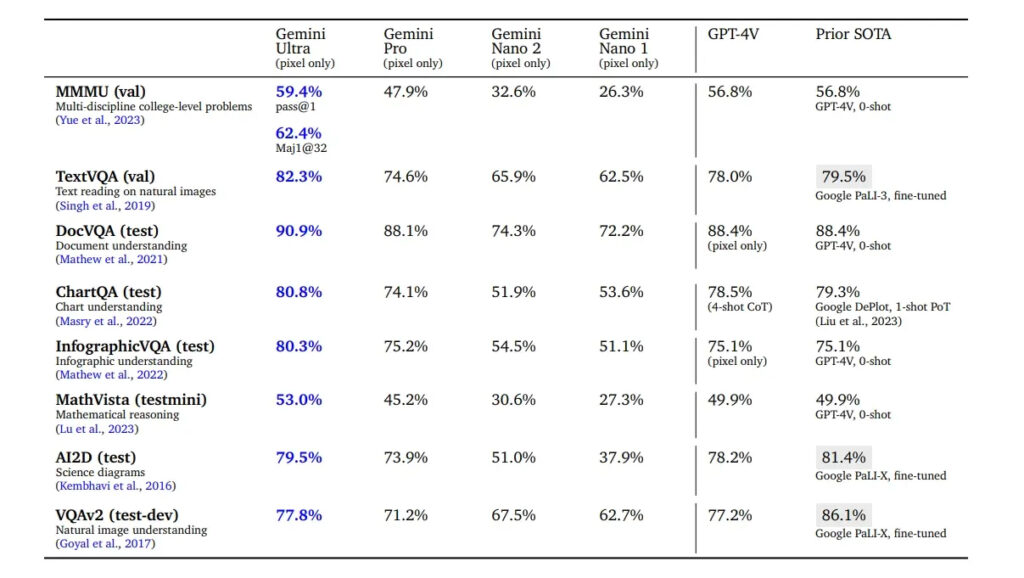
For a comprehensive analysis between Gemini Ultra and GPT-4, further insights are available in the research paper released by Google Deepmind. These benchmark numbers affirm Google’s successful development of a competitive model capable of rivalling top-tier Large Language Models, notably GPT-4. Moreover, in terms of multimodal capabilities, Google has showcased a significant advancement, reaffirming its prowess in the field.
Gemini AI: Safety Checks in Place
Google, in alignment with its commitment to bold and responsible AI development, upholds rigorous safety measures within its Google Deepmind team. Before public release, Google ensures thorough internal and external testing of its models.
Implementing proactive policies for Gemini models, Google actively screens for biases and toxicity in user input and responses, significantly reducing instances of model hallucination. Collaborating with external entities like MLCommons, Google conducts red-team evaluations to assess AI system integrity. Additionally, Google is spearheading the creation of a Secure AI Framework (SAIF) for the industry, aimed at addressing risks inherent in AI systems.
Currently, Google is conducting comprehensive safety assessments for its potent Gemini Ultra model, slated for an early release next year after the completion of these meticulous safety checks.
Verdict: The Gemini AI Era is Here
While Google was initially caught off guard by the release of ChatGPT a year ago, it appears that the development of the Gemini models has brought them on par with OpenAI. The Ultra model, in particular, stands out as impressive, and we’re eager to test it despite some questionable benchmark results. Its remarkable visual multimodal capabilities and top-notch coding performance, as highlighted in the research paper, are quite promising.
The Gemini models signal a departure from Google’s previous AI endeavors, bearing a distinct feel of AI systems crafted from the scratch. However, there’s anticipation that OpenAI might counter with GPT-5 upon the release of Google’s Gemini Ultra model early next year, setting the stage for a competitive race. What are your thoughts on Google’s latest Gemini AI models? Do express your thoughts in the comments section!
0 Comments